WiFi Pineapple Mark VII – Expanding the Workflow with Automation and Device Profiling
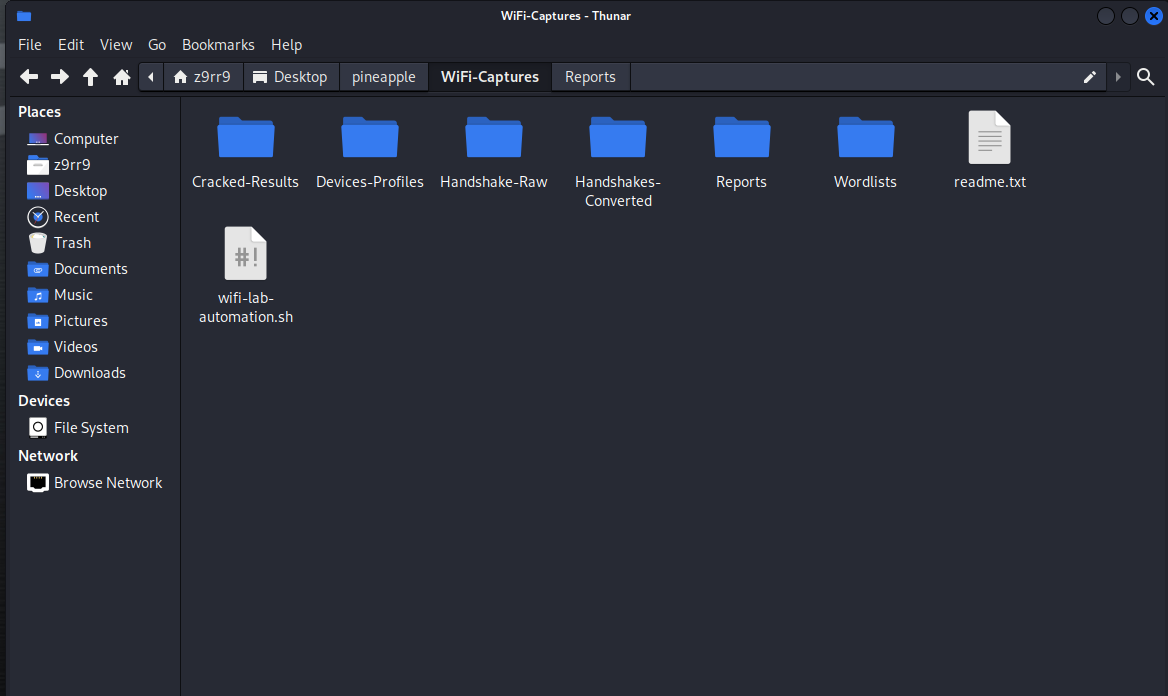
As I continued working with the WiFi Pineapple, I wanted to bring my lab environment to a much more structured and automated level.
Collecting handshakes and running campaigns is already a good foundation, but the goal was to streamline the entire process — from gathering data to analyzing and documenting it in one flow.
After running several longer campaigns and observing the Pineapple's behavior over time, I noticed that while campaigns are active, the Pineapple writes continuously to the report files. These reports are saved as both JSON and HTML formats. The JSON files are especially interesting because they include structured data about devices, timestamps, signal strengths, and sometimes even vendor information. Perfect for automation.
To avoid manual file handling between the Pineapple and my Kali lab environment, I developed a dedicated synchronization script. This script connects via SSH to the Pineapple and automatically pulls the latest campaign reports from all campaign folders into my working directory on Kali. It checks for both .json and .html files and lists out the results at the end, so I always know exactly what has been transferred.
A snippet of the sync logic looks like this:
REMOTE_DIR="/root/loot/campaigns/"
LOCAL_DIR="/path/to/lab/Reports/"
scp root@pineapple_ip:"${REMOTE_DIR}"*.json "$LOCAL_DIR"
scp root@pineapple_ip:"${REMOTE_DIR}"*.html "$LOCAL_DIR"
An important learning during this step was understanding the timing: If I sync the reports while the campaign is still running, the JSON files are technically incomplete because the Pineapple is still writing data into them. Especially the clients section in the report can still be empty at that point. However, with my updated automation scripts, this is not an issue anymore. The script now checks whether the clients array exists and skips empty or incomplete reports gracefully. This way, even if I sync during an active campaign, the process doesn't break, and the profiles remain clean.
Here’s how the device profile generator checks for valid data in the report:
has_clients=$(jq 'has("clients") and (.clients != null)' "$report")
if [[ "$has_clients" != "true" ]]; then
echo "No clients found in report: skipping."
continue
fi
After syncing, the next step is generating device profiles based on the reports. For this, I created a dedicated profile generation script that reads through the JSON files and creates individual text-based profiles for each device observed. These profiles include valuable information like:
- The device MAC address
- Manufacturer (if available)
- First and last seen timestamps
- Observed SSIDs
- The report from which the data was extracted
For each device found in the report, the script automatically creates a profile file in the lab folder. A basic structure of the generated profile looks like this:
Device: 00:11:22:33:44:55
Manufacturer: ExampleVendor Inc.
First Seen: 2025-04-07T08:12:34Z
Last Seen: 2025-04-07T08:45:12Z
Observed SSIDs: HomeWiFi, GuestNetwork
Observed in report: campaign-2025-04-07.json
Parallel to these improvements, I also thought ahead to the password cracking phase. To prepare properly, I wanted to create tailored wordlists for different router types, especially for commonly used models like FRITZ!Box and others. Instead of relying purely on pre-made wordlists, I automated the generation of custom lists using Crunch, a powerful wordlist generation tool.
For example, I created a script that automatically generates patterns for FRITZ!Box default passwords. These routers often use uppercase letters and digits in a specific length, so the script looks something like this:
#!/bin/bash
# Generate FRITZ!Box style wordlist
crunch 16 16 -o fritzbox-wordlist.txt -t @@%%@@%%@@%%@@%%
This command builds a pattern where @ represents uppercase letters and % represents numbers, perfectly fitting the typical structure of FRITZ!Box passwords.
To make the workflow more flexible, I also prepared scripts for other router types, adjusting the patterns and lengths accordingly. Each router model has its own script and a README file for clarity, ensuring that I can easily expand the collection in the future without losing track.
Example structure for such a README:
README - Wordlist Generator for FRITZ!Box
Description:
Generates a wordlist for FRITZ!Box default password patterns using Crunch.
Pattern used:
@@%%@@%%@@%%@@%%
Usage:
chmod +x generate-fritzbox-wordlist.sh
./generate-fritzbox-wordlist.sh
Output:
fritzbox-wordlist.txt
With these automatic generators in place, I save a lot of time compared to manually creating lists or relying on huge, unfocused wordlists. More importantly, I can now create router-specific lists that are both smaller in size and more precise, increasing efficiency during the cracking phase.
I also made sure to maintain proper documentation for each script and process. Every tool in my lab now has an accompanying README file, outlining its purpose, usage, and dependencies. For example, I noted the dependency on jq for processing JSON files:
sudo apt install jq
This way, I ensure that the setup remains reproducible, and should I need to rebuild my environment in the future, everything is clearly documented.
One of the biggest improvements is the clean separation of tasks:
- The Pineapple handles the scanning and data collection.
- My sync script reliably pulls the latest reports.
- The profile generation script processes these reports and updates the device profiles.
- The wordlist generators prepare focused attack lists based on router models.
- I maintain a well-organized folder structure for raw data, processed results, wordlists, and documentation.
Through this process, I’ve created a workflow that not only feels professional but also scalable. Step by step, I am transforming my initial exploratory lab into a real pentesting environment that documents, automates, and evolves over time.
Additionally, one of the technical challenges I wanted to solve was the repetitive SSH password prompt when syncing files from the Pineapple. To resolve this, I developed an automated script that sets up SSH key-based authentication. Now, my Kali machine connects to the Pineapple securely without asking for a password each time. The script not only copies my SSH key to the Pineapple but also configures it persistently by adding the setup into the Pineapple's startup routine. This ensures that even after a reboot, the key remains active, and I don’t have to repeat the setup.
The automation script for this looks like:
#!/bin/bash
# Automated SSH key setup for Pineapple
PINEAPPLE_IP="172.16.42.1"
LOCAL_PUB_KEY=$(cat ~/.ssh/id_rsa.pub)
ssh root@"$PINEAPPLE_IP" << EOF
mkdir -p /root/.ssh
chmod 700 /root/.ssh
echo "$LOCAL_PUB_KEY" > /root/.ssh/authorized_keys
chmod 600 /root/.ssh/authorized_keys
# Ensure persistence via rc.local
touch /etc/rc.local
chmod +x /etc/rc.local
if ! grep -q "echo \"$LOCAL_PUB_KEY\"" /etc/rc.local; then
sed -i '/^exit 0/i mkdir -p /root/.ssh' /etc/rc.local
sed -i "/^exit 0/i echo \"$LOCAL_PUB_KEY\" > /root/.ssh/authorized_keys" /etc/rc.local
sed -i '/^exit 0/i chmod 700 /root/.ssh' /etc/rc.local
sed -i '/^exit 0/i chmod 600 /root/.ssh/authorized_keys' /etc/rc.local
fi
EOF
ssh root@"$PINEAPPLE_IP" "reboot"
This small improvement alone streamlined my workflow significantly, as I no longer have to enter my password multiple times during each synchronization or automation task.
With these building blocks in place, I also took the time to clean up my entire project structure. All scripts are now organized within clearly defined folders, each accompanied by a README file explaining their usage and purpose. This structure ensures that as my lab grows, I maintain clarity and ease of use.
The setup now feels like a proper pentesting toolkit. I know exactly where to find my raw handshakes, processed device profiles, generated wordlists, and campaign reports. Each step from data collection to analysis and attack preparation is covered by its own script, making the entire process efficient and professional.
Next, I plan to further optimize my environment by potentially chaining my scripts together. This way, I could automate the complete cycle: campaign completion triggers the sync, which in turn automatically starts profile generation and prepares data for cracking. Such improvements will continue to bring me closer to a fully automated wireless pentesting lab that not only saves time but also strengthens my understanding of operational workflows in real-world scenarios.